Welcome
The LPSM is a research unit jointly supported by CNRS, Sorbonne Université and Université Paris Cité. The unit hosts about 200 members (about 90 faculty) and is located at two sites (Campus P. et M. Curie of Sorbonne Université et Campus Paris Rive Gauche of Université Paris Cité).
The LPSM research activities cover a broad spectrum in Probability and Statistics, from the most fundamental aspects (which, in particular, include Stochastic Analysis, Random Geometry, Numerical Probabilities and Dynamical Systems) to applications in the Modelling in various disciplines (Physics, Biology, Data Sciences, Finance, Insurance, etc). Applications involve partnerships with the non-academic sector.
While the unit LPSM is relatively recent, its components have deep roots in the rich history of the “mathematics of randomness” that has unfolded in Paris during the 20th century (see here for more details).
NB: This website is largely inspired by the one of IRIF.
News
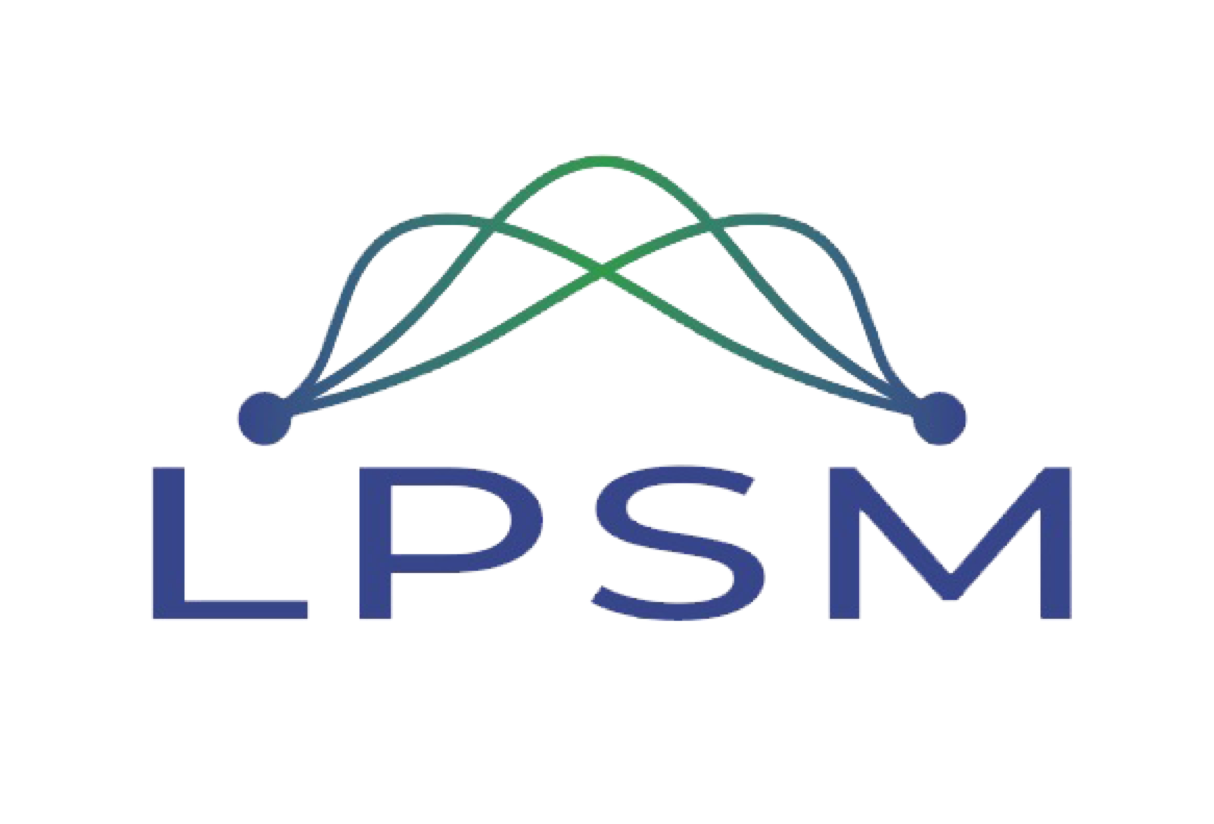
12.9.2024
Lancement du séminaire sur les processus de Hawkes, qui aura lieu les mardis à 11h sur le site de Jussieu. Programme ici.
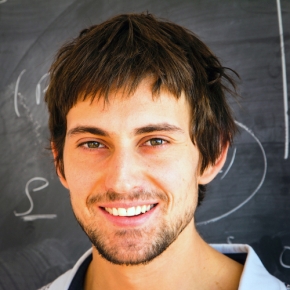
9.9.2024
Le projet “New algebraic structures in quantum integrability: towards 3D” porté par Eric Vernier à reçu un financement JCJC de l'ANR, ainsi qu'un financement Emergence de l'Université Paris Cité.
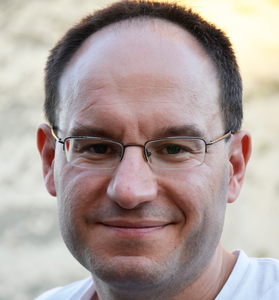
9.9.2024
Lorenzo Zambotti est lauréat du Prix Frontiers of Science pour son article Algebraic renormalisation of regularity structures paru dans Inventiones Mathematicae. Félicitations à Lorenzo!
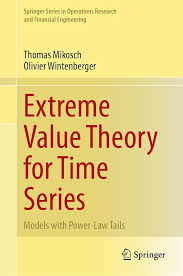
4.10.2024
Le livre Extreme Value Theory for Time Series, écrit par Thomas Mikosch et Olivier Wintenberger, vient d'être publié.
(Ces actualités sont présentées selon un classement mêlant priorité et aléatoire.)
Events
Séminaire Modélisation et Probabilités
Mercredi 23 octobre 2024, 14 heures 15, Sophie Germain 1016
Barbara Dembin (IRMA Strasbourg) Surfaces minimales en environnement aléatoire
Séminaire de Probabilités
Mardi 5 novembre 2024, 14 heures, Jussieu, Salle Paul Lévy, 16-26 209
Cécile Mailler (University of Bath) A venir
Séminaire du LPSM
Jeudi 7 novembre 2024, 9 heures 30, Amphi Durand - Jussieu
Rémi Bardenet A préciser
Séminaire sur les processus de Hawkes
Mardi 12 novembre 2024, 14 heures, Jussieu, Salle Emile Borel, 15-26 201
Stefano Spaziani (Université de Nice) Heterogenous multiscale multivariate autoregressive model, sparse estimation and application in neuroscience
Séminaire de Probabilités
Mardi 19 novembre 2024, 14 heures, Jussieu, Salle Paul Lévy, 16-26 209
Marie Albenque (IRIF, Université Paris Cité) A venir